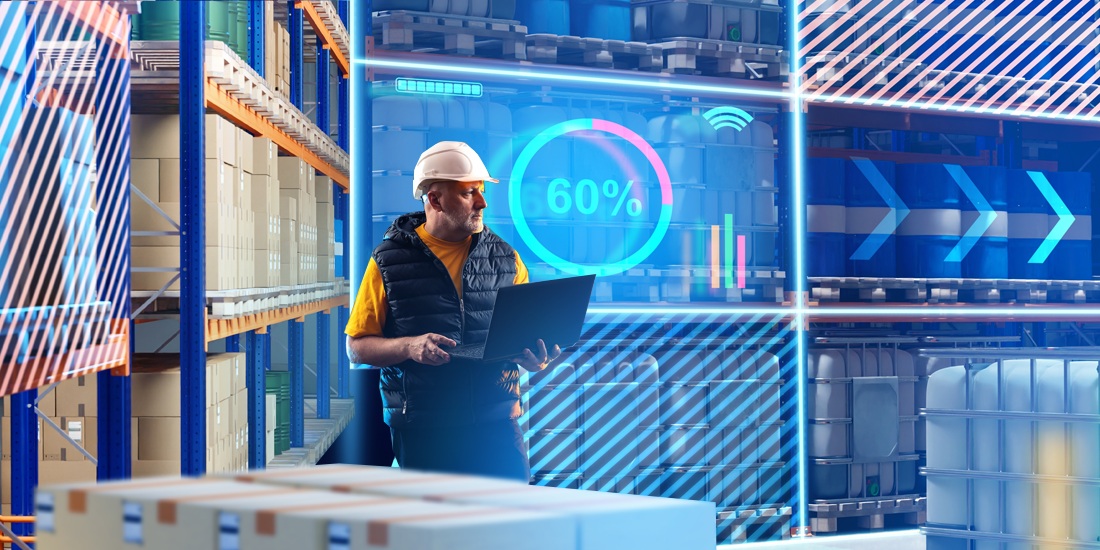
Machine learning (ML) and artificial intelligence (AI) are transforming supply chain forecasting from an art to a science
In this blog, we’ll explore how – as well as present common benefits and challenges, real-like case studies from international corporations, predictions for future trends – and how ICRON leverages ML and AI in its supply chain management solution.
What is Forecast Accuracy in Supply Chain Operations?
Understanding Forecast Accuracy
Forecast accuracy refers to the precision with which businesses can predict future demand for their products or services. It measures how closely projected demand aligns with actual demand.
In supply chain operations, forecast accuracy directly impacts:
- Inventory management
- Production planning
- Resource allocation
- Customer satisfaction
- Profitability
This is because high forecast accuracy allows organizations to maintain optimal inventory levels, reduce carrying costs, minimize stockouts, and make informed decisions about procurement, manufacturing, and distribution.
The Traditional Challenges of Forecasting
Traditional forecasting methods rely heavily on historical data and basic statistical models. These approaches often fall short in today’s for several reasons:
- They struggle to account for numerous variables and complex relationships between factors that affect demand
- They’re unable to rapidly adapt to sudden market shifts or unexpected events
- They fail to incorporate external factors like economic indicators or weather patterns
- They require a significant amount of manual intervention
- They can’t efficiently process large volumes of data
How Machine Learning and AI Improve Forecast Accuracy
ML and AI work together to create highly accurate demand forecasts. Here’s a look at how.
Data-Driven Decision Making
ML and AI technologies can easily process large amounts of data from many different sources, creating actionable insights that drive accurate forecasts.
ML algorithms, for example, can analyze structured and unstructured data to identify patterns and relationships that humans might miss.
Meanwhile, AI systems can simultaneously analyze internal data – such as sales history, inventory levels, and production capacity – alongside external factors like market trends, and competitor actions to create a holistic view of demand drivers.
Predictive Analytics for Demand Forecasting
ML models use predictive analytics techniques that reduce the likelihood of forecasting errors by as much as 50%. [1]
These techniques include:
- Time series analysis. Algorithms like ARIMA (autoregressive integrated moving average) and Prophet can identify seasonal patterns, trends, and cycles in historical data to project future demand
- Regression models. Machine learning can determine relationships between multiple variables – including price, promotions and competitor actions – and quantify their impact on demand
- Neural networks. Deep learning models can detect complex, non-linear relationships and hidden patterns in data, which is very effective for products with fluctuating demand patterns
- Ensemble methods. By combining multiple forecasting techniques, AI can leverage the strengths of different approaches for highly accurate predictions
Real-Time Adjustments and Adaptive Forecasting
One of the most powerful advantages of ML-based forecasting is its ability to continuously learn and adapt. As new data becomes available, machine learning models automatically adjust their predictions, using the latest information without manual intervention. This can be extremely valuable when responding to unexpected events or rapid market changes.
For example, if a competitor cuts prices or there is a sudden shift in customer orders, AI systems can quickly analyze the impact and adjust production or inventory forecasts. This helps manufacturers respond to changes in demand before they cause delays or overstock.
Improving Lead Time and Reducing Stockouts
By improving forecast accuracy, ML and AI can positively impact lead time and stockout rates. More precise demand predictions enable organizations to optimize order quantities and timing, so products are always available when needed without excessive inventory.
AI can also identify early warning signals of potential stockouts by monitoring demand patterns, supplier performance, and logistics data. When the system detects a heightened risk, it can trigger proactive measures to prevent disruptions, such as expediting shipments or sourcing from alternative suppliers.
The Benefits of Using Machine Learning and AI for Forecasting
ML and AI result in numerous benefits for organizations that implement them into their demand forecasting strategies.
Enhanced Operational Efficiency
With more accurate forecasts, you can optimize your entire supply chain. A well-implemented ML and AI-drive demand forecasting strategy can result in:
- Reduced excess inventory and associated carrying costs
- Decreased waste from obsolete or expired products
- Optimized production scheduling and resource utilization [2]
- More efficient transportation and logistics planning
- Lower emergency shipping costs and expediting fees
Improved Inventory Management
AI-driven forecasting drives proactive inventory management. Organizations can maintain optimal stock levels across multiple sites or facilities to balance the risk of stockouts against the costs of excess inventory.
Additionally, ML can enable increasingly granular inventory optimization, so businesses can take different approaches to inventory management based on
- Product characteristics
- Product location
- Customer requirements
This is particularly valuable for companies managing thousands of SKUs or product types in multiple locations.
Faster and Smarter Decision-Making
AI allows you to quickly make informed decisions. Instead of spending hours analyzing data, employees can focus on strategic initiatives and big-picture processes, while automated systems handle routine tasks.
AI can also simulate different scenarios and recommend the best courses of action. For example, an AI system might suggest reallocating inventory between locations, or adjusting production schedules to address projected demand changes.
Greater Flexibility and Responsiveness
In supply chain management, the ability to respond quickly to change is often the difference between success and failure. AI-enhanced forecasting provides the agility needed to effectively navigate such disruptions.
By continuously monitoring signals from the market and supply chain, ML models help organizations anticipate challenges and opportunities earlier. This allows businesses to pivot strategies, reallocate resources, or implement contingency plans before disruptions occur.
Challenges in Implementing AI and ML for Forecasting
As with any technology-driven tools, AI and ML pose substantial challenges to organizations seeking to implement them.
Data Quality and Availability
Challenges surrounding data and data quality are a huge reason why many AI-driven projects are delayed – or even fail entirely. [3] Organizations implementing AI for demand forecasting often face data-related challenges caused by:
- Inconsistent or incomplete historical data
- Data silos across different departments or systems
- Unintegrated internal and external data sources
- Poor data governance practices
- Limited visibility into supplier or customer data
Integration with Legacy Systems
Many organizations operate with established ERP systems or legacy forecasting tools that weren’t designed with AI integration in mind [4]. This creates complications for AI’s implementation, especially regarding:
- Data extraction and transformation
- Real-time information flows
- System performance and scalability
- Maintaining data integrity across platforms
Cost and Resource Requirements
Implementing AI forecasting solutions typically arrives with substantial financial cost, especially for enterprise-level organizations [5], which must invest in the technology itself, as well as change management and training expenses that go along with it.
Cost factors to consider here include:
- Software licenses or development costs
- Technical infrastructure requirements
- Data scientists and ML engineers
- Training or upskilling for existing staff
- Ongoing maintenance and model refinement
Best Practices for Implementing AI/ML for Forecasting
We have noticed several best practices organizations that successfully implement AI and ML solutions tend to use. We’ll outline them here.
Data Collection and Preparation
Success in demand forecasting begins with a solid foundation of data. To build one, you’ll need to take a multifaceted approach – one which starts with auditing existing sources of data and identifying gaps.
You should also consider establishing procedures and processes for data governance and data standardization procedures, creating a unified data repository, and developing methods to incorporate external data.
Continuous Learning and Model Refinement
AI implementation isn’t a one-time project but an ongoing process that requires refinement and improvement. You’ll need to regulatory monitor your AI or ML model – as well as adjust them as business needs or market conditions shift. You should also retrain your models data when and where relevant, as well as test alternative algorithms and approaches, so you can find the best fit.
Cross-Department Collaboration
Successful AI implementation requires alignment across multiple functions, including stakeholders from departments like sales, marketing, operations, finance, and IT. Creating cross-functional teams is an effective method of accomplishing this. You can also establish shared KPIs and success metrics, and collaborative processes for forecast review and adjustment.
Real-World Examples of AI and Machine Learning Improving Forecasting
Here are a few examples of enterprise-level organizations that have implemented AI and ML-powered forecasting strategies to great effect.
Case Study: Amazon’s Demand Forecasting
Amazon has revolutionized inventory management with a sophisticated application of machine learning via its AWS platform. [6]
Amazon’ forecasting system analyzes billions of data points daily, including historical sales, product attributes, website browsing patterns, planned promotions, and even weather forecasts. This helps the company manage inventory of more than 400 million products in nearly 200 countries.
Case Study: Coca-Cola’s AI-Driven Demand Planning
Coca-Cola faces complex forecasting challenges with numerous products, seasonal demand patterns, and global distribution.
To approach these challenges in Japan, the company uses an AI-powered demand planning system that analyzes data from sales, marketing campaigns, weather patterns, local events, and economic indicators. [7]
Future Trends in AI and ML for Supply Chain Forecasting
The following are trends that are already rising in popularity due to their advantageous effect on supply chain efficiency, agility, and decision-making. We expect them to continue to do so.
Evolution of AI Algorithms
AI will continues to advance rapidly, with promising developments for supply chain forecasting. These include:
- Deep learning. More sophisticated neural network architectures will enable even more accurate predictions from complex data
- Reinforcement learning. Systems that learn optimal inventory policies through trial and error will revolutionize replenishment strategies
- Causal AI. Moving beyond correlation to understand true cause-and-effect relationships will produce increasingly robust forecasts
- Explainable AI: Advancements in making AI decisions more transparent will increase trust and adoption
Integration with IoT and Real-Time Data
The proliferation of Internet of Things (IoT) devices – with 40 billion IoT devices expected by 2030 [8] – is creating new opportunities for real-time supply chain visibility. Use cases here include:
- Smart shelves that can automatically track inventory levels
- Connected vehicles with precise location and condition monitoring
- Manufacturing equipment that can instantly share production data
- Consumer devices offering insights into usage patterns and product performance
Collaboration and Ecosystem Integration
The future of forecasting lies in collaborative approaches that span organizational boundaries, such as:
- Shared forecasting platforms between retailers and suppliers
- Industry-wide data standards and exchange protocols
- Blockchain-based systems for secure data sharing
- AI-enhanced collaborative planning, forecasting, and replenishment (CPFR)
Unlock AI-Driven Supply Chain Optimization with ICRON
ICRON’s AI & ML capabilities help you identify patterns, predict outcomes, and automate decisions across your supply chain – giving you the edge to stay ahead of disruptions.
Want to see how AI can make your supply chain smarter and more resilient?
References
- https://www.mckinsey.com/capabilities/operations/our-insights/supply-chain-40--the-next-generation-digital-supply-chain
- https://www.deloitte.com/ch/en/Industries/technology/perspectives/artificial-intelligence-Is-changing-how-companies-work.html
- https://www.ibm.com/thought-leadership/institute-business-value/en-us/report/ai-data-integration
- https://papers.ssrn.com/sol3/papers.cfm?abstract_id=5179431
- https://www.forbes.com/councils/forbestechcouncil/2023/08/31/the-hidden-costs-of-implementing-ai-in-enterprise/
- https://www.amazon.science/latest-news/the-history-of-amazons-forecasting-algorithm
- https://supplychaindigital.com/operations/coca-cola-bottlers-japan-selected-o9-supply-optimisation
- https://iot-analytics.com/number-connected-iot-devices/