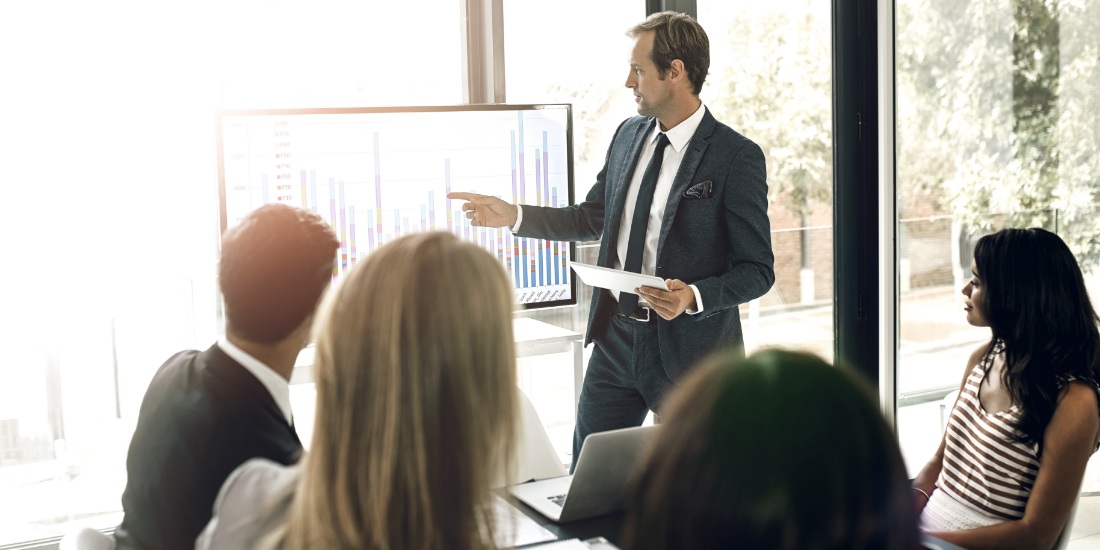
Demand forecasting improves supply chain efficiency by anticipating market fluctuations or changing customer expectations – allowing you to respond on time.
In this article, we’ll explore the definition of demand forecasting and its importance in business operations. We’ll also touch on its benefits, challenges, best practices, and future trends – as well as how ICRON can help you use demand forecasting to optimize your organization’s supply chain.
Understanding Demand Forecasting
What is Demand Forecasting?
Demand forecasting involves using gathered data, market intelligence, statistical techniques, and predictive analytics to estimate future customer demand for products or services.
It goes beyond guesswork, with business leaders using technology to make better, more nuanced predictions and improve the supply chain.
Demand forecasting typically encompasses:
- Collecting and analyzing sales data
- Identifying patterns and trends
- Incorporating external market factors
- Using statistical and machine learning (ML) models
- Generating predictions of future product or service demand
Importance of Demand Forecasting in Business Operations
Demand forecasting is an extremely important tool that influences nearly every aspect of business operations – as well as every aspect of the supply chain.
It offers a data-driven foundation for:
- Inventory management. Precise demand forecasting allows businesses to maintain optimal inventory levels, reducing the risks of stockouts and the costly burden of excess inventory
- Production planning. Manufacturers can use demand forecasting to optimize production schedules, effectively allocate resources, and reduce downtime and bottlenecks.
- S&OP planning. Demand forecasts provide crucial inputs for financial modeling, enabling more accurate and effective revenue projections, budget guidelines, and risk management strategies
- Supply chain optimization. With a clear idea of future demand for products , businesses can negotiable better terms with suppliers, refine procurement strategies, and reduce lead times and supply chain disruptions
Methods of Demand Forecasting
A well-designed demand forecasting strategy involves both qualitative and quantitative methods of data collection. In this section, we’ll take a look at each, as well as spotlight the popularity of a hybrid approach to supply chain demand forecasting.
Qualitative Methods
Qualitative methods of data collection for demand forecasting rely on expert opinions and market research. They provide valuable insights when hard data is limited or non-existent.
For example, techniques such as the Delphi method [1] involve consulting industry experts to gather informed perspectives. Additionally, organizations often ask their in-house sales teams to provide them with market insights. This allows them to assemble an accurate image of potential customer demand.
Quantitative Methods
Quantitative methods, on the other hand, use statistical models to analyze numerical data. Time series analysis, for instance, examines past demand patterns to forecast future trends, while causal models examine the relationship between demand and factors like economic indicators or marketing campaigns.
Machine learning tools are also becoming increasingly important in demand forecasting, with 80% of business leaders having already implemented or planning to implement them in their supply chain management strategies. [2]
ML tools can analyze large sets of data to find patterns traditional methods might miss and develop predictions that are more accurate. They can also adapt to new data and continuously improve their accuracy over time, making them particularly valuable in dynamic markets.
Hybrid Approaches to Demand Forecasting
Companies that are looking to optimize their demand forecasting methods typically combine qualitative and quantitative methods. This allows them to holistically approach their demand forecasting strategy to be sure that it is as accurate as possible.
A manufacturer might use a quantitative approach by analyzing past sales data, production capacity, and supplier lead times to predict demand for a product. At the same time, they could apply a qualitative approach by consulting with suppliers about potential raw material shortages and gathering insights from dealers on customer preferences.
Benefits of Demand Forecasting in Operational Optimization
A well-implemented demand forecasting strategy has wide-ranging benefits that affect every point of the supply chain. Let’s take a look.
Enhanced Inventory Management
Demand forecasting helps businesses maintain optimal inventory levels by predicting future demand patterns. This will help your organization achieve results like:
- Minimized excess inventory holding costs
- Reduced risk of stockouts
- Optimized warehouse space utilization
- Improved cash flow by aligning inventory investments with anticipated demand
- Decreased waste associated with overstocking
Improved Production Planning
Accurate demand forecasting helps organizations align production schedules with expected demand. In turn, this can lead to more efficient business and supply chain operations by:
- Aligning production capacity with anticipated market demand
- Reducing manufacturing downtime
- Optimizing resource allocation
- Minimizing production waste
- Improving overall equipment effectiveness (OEE)
- Enabling more flexible and agile manufacturing processes
Optimized Workforce Management
A demand planning strategy allows you to predict demand fluctuations in the supply chain, which can help team leaders schedule staff to match workload. This prevents both under- and overstaffing, allowing you to maintain an efficient workflow while keeping staff-related costs in check.
Better Financial Planning
Reliable demand forecasts support more accurate revenue projections and budgeting. You’ll be able to efficiently allocate resources, better manage cash flow, and make informed investment decisions based on anticipated sales trends.
Challenges in Demand Forecasting
Despite its advantages, demand forecasting also presents several complex challenges to organizations. Here are a few of the most common, as well as strategies you can use to navigate them.
Data Quality and Availability
Effective demand forecasting relies on high-quality, comprehensive data. However, that is easier said than done. Inconsistent or incomplete historical data – or siloed information across different departments or facilities – can result in inaccuracies and inefficiencies. It may also be difficult to collect external market data, depending on your industry or sector.
By standardizing your methods of data collection – then creating centralized location for its storage – you can mitigate data-quality-related challenges. Should you run into difficulties finding external data, you can use multiple sources, such as industry reports, public databases, and supplier-collected information.
Market Volatility
Shifting markets pose a substantial challenge to supply chain organizations. This volatility is often caused by:
- Technological changes
- Unexpected economic shifts
- Shifts in consumer behavior
- Geopolitical events affecting supply chains
To effectively approach market volatility, organizations can adopt agile supply chain strategies [3], use real-time data analytics, and implement scenario planning to quickly adapt to market changes and minimize risk.
Technological Integration
When using an advanced demand forecasting strategy, you may face multiple technological challenges, including compatibility issues with legacy systems and complex data migration processes.
Here, you can consider adopting a phased implementation plan, as well as providing employees with deep training into any new tools they’ll need to use, so you can seamlessly ease into your demand forecasting strategy.
Best Practices for Effective Demand Forecasting
Utilize Advanced Analytics and AI
New technologies are transforming demand forecasting capabilities that can help organizations in myriad ways. For example, AI-driven demand forecasting can help organizations realize a 30% reduction in excess inventory. [4]
Other potential use cases include:
- ML tools for demand pattern recognition
- Neural networks for complex predictive modeling
- Automated anomaly detection
- Continuous model self-improvement
Collaborate Across Departments
As with any supply chain strategy, successful demand forecasting requires a holistic, cross-functional approach that includes multiple stakeholders.
The most accurate demand forecasting requires organizations to break down organizational siloes, create clear communication channels, and develop single-source-of-truth methods of tracking, gathering, and sharing data across multiple facilities, departments, and teams.
Continuous Monitoring and Adjustment
Effective demand forecasting requires continuous refinement through regular performance evaluations and feedback.
Developing flexible forecasting frameworks and establishing KPIs to measure accuracy is key here, as such they can help ensure that your demand forecasting methods remain responsive and reliable in the face of changing market conditions.
Future Trends in Demand Forecasting
Here are a few of the trends that we predict will shape the way demand forecasting is used in the near future.
Real-Time Data Integration
New technologies – such as IoT sensors, social media sentiment analysis, and real-time market trend tracking – are transforming data-driven forecasting. These tools can instantly process data and use predictive analytics that allow you to react quickly to shifts in demand.
Predictive Analytics and Big Data
Advanced analytics and big data are reshaping demand forecasting with capabilities like ML, multi-variable analysis, and probabilistic models. Innovations like quantum computing [5] and hyper-personalized predictions will continue to help businesses anticipate demand with greater precision and nuance.
Collaborative Forecasting Platforms
Demand forecasting will increasingly rely on interconnected, intelligent systems like cloud-based platforms, blockchain-enabled transparency [6], and AI-driven synchronization. Together, these systems aim to improve supplier-retailer collaboration through automated communication and international networks for demand prediction.
Drive Accurate Demand Forecasting with ICRON Demand
ICRON Demand uses AI, decision intelligence, and collaborative analytics to help supply chain managers make smarter choices.
With ICRON Demand, you can:
- Predict demand using historical data, market trends, and sales targets
- Identify key focus areas with data-driven insights.
- Optimize resource allocation, budgeting, and performance tracking
- Cuts inventory costs and spoilage
- Boost visibility and control for complex, multi-region businesses
Ready to get started? Get in touch today!
References
- https://www.sciencedirect.com/topics/social-sciences/delphi-method
- https://www.mckinsey.com/capabilities/operations/our-insights/to-improve-your-supply-chain-modernize-your-supply-chain-it
- https://www2.deloitte.com/us/en/insights/industry/manufacturing/global-supply-chain-resilience-amid-disruptions.html
- https://wjarr.com/sites/default/files/WJARR-2024-2394.pdf
- https://www.ibm.com/thought-leadership/institute-business-value/en-us/report/quantum-computing-supplychain
- https://thefinancialexpress.com.bd/views/views/how-blockchain-technology-can-facilitate-supply-chain-and-logistics-1562511042