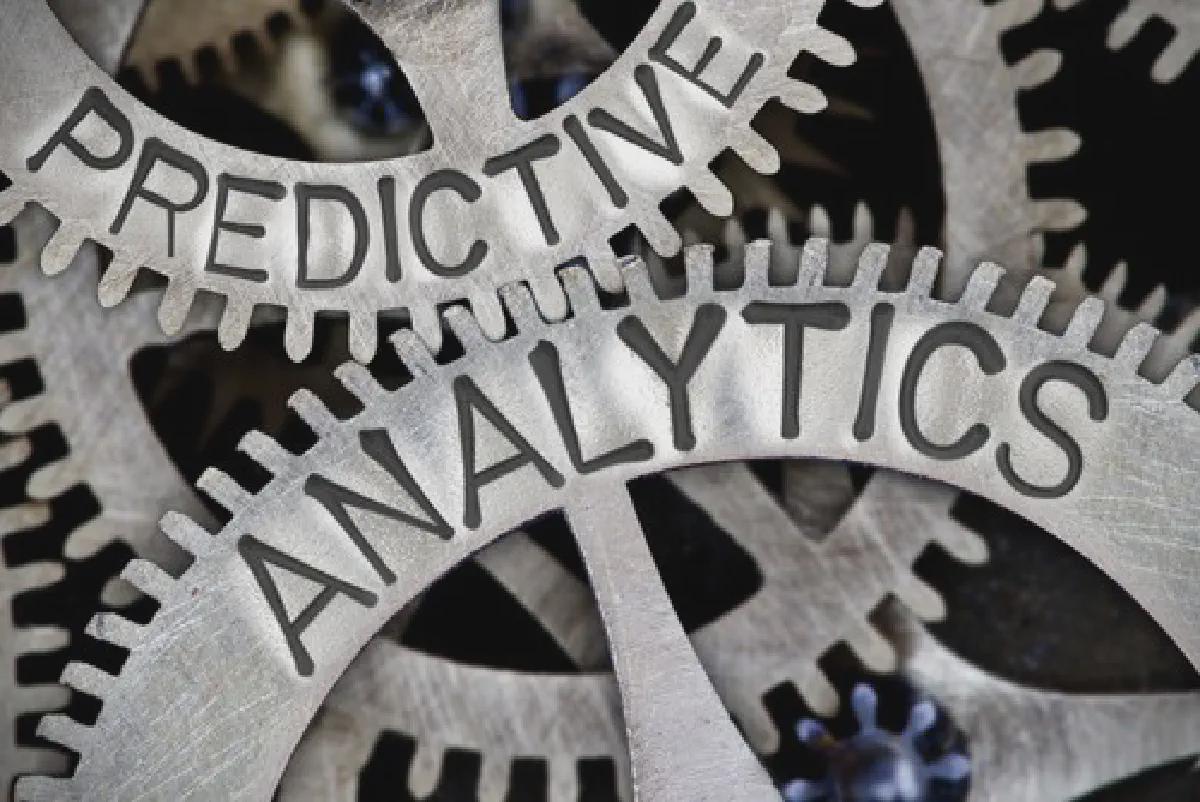
Author: Dr.ir. Arjen Vestjens | Managing Partner CQM
Forecasting is predicting the future with the help of quantitative methods. Crucial for many organizations. Because, if you know what happens in the future, you can already respond to it. But which technique do offer the most reliable prediction? The classic method with formulas and transparency? Or do you enthusiastically dive into the new world of the deep learning network? ICRON’s partner CQM gives you an clear insight into predictive analytics.
How many passengers are there in the train next year? How big will the demand for my products be next quarter? And how much mail should be processed in my sorting center next week? A good insight into the future helps organizations to make the right decisions in time. They can better plan their activities and reserve sufficient people and resources. This way they keep their processes efficient and their customers happy. Forecasting is the quantitative way to make predictions. Historical data and process knowledge form the largest part of the input. Predictive analytics techniques recognize the patterns, make the connections and can make predictions.
Classic vs modern
Nowadays there are many techniques for predictive analytics. Overall they can be divided into two groups. First, the classical methods, usually called regression analysis techniques. Based on historical data and domain knowledge (such as the subject-specific knowledge at the customer), quantitative formulas are built to make connections, give weight to individual factors and then make the predictions. There are many such techniques with their own specific applications.
This classic method provides optimal control and transparency: every outcome can be explained. On the other hand, there is the sensation of this moment: deep learning, a part of machine learning. A machine (computer) is fed with numerous learning examples. These are various data sets of input and outcome. The more examples the machine receives, the better it will be in recognizing patterns and making predictions for new situations. A fantastic technology in the new world of artificial intelligence. There is one problem, however: there is a black box between feeding learning examples and getting predictions. Nobody knows exactly how the machine has reached the relevant predictions. Moreover, the quality of the learning examples must also be of impeccable and unbiased quality. Otherwise, mistakes or prejudices are simply included in the system.
Choice
On the one hand, it is quite nice to stick to traditional methods with formulas that offer total control. But the potential of artificial neural networks that train themselves with smart algorithms to discover patterns and find valuable information (in short: deep learning) is unprecedented. Especially with large amounts of unstructured data such as applications for image recognition, speech recognition and text analysis. Research has shown that deep learning networks perform comparable or even better than the human brain for those complex applications. They are also well applicable in forecasting. For example, CQM has predicted the number of visitors at “Paleis Het Loo” (Dutch Royal Palace) with classic statistical regression methods, because the customer wanted insight into the driving factors.
As a trial, CQM also set up and trained a deep learning network for this specific situation. The predictions of both methods were equivalent. In addition, CQM has the knowledge to make the black box of deep learning a bit less black. With techniques such as Pattern Recognition Feed forward Network or the in-house developed COMPACT CQM can gain more insight into why the neural network advises a certain decision or has made a prediction.
Balance
Does the choice obviously fall on modern deep learning? No, not necessarily. The temptation is great to surf the hype, but the interests of organizations are often too big. It is still the people who have to do it. First determining the best method for a specific situation. For one situation, deep learning is ideal; for the other, a controlled regression method is the most suitable. This requires knowledge of the old and the new techniques. Then it is again people who make the choices for the input for forecasting, formulas for regression or training for deep learning. This requires not only extensive expertise from mathematicians and statisticians, but also domain knowledge at the customer. And this applies equally to the interpretation of decisions and predictions made by classical or modern technology. That is why CQM always starts with that practical expert knowledge. Professional forecasting is therefore never a simple choice for an old or new technique. It is a nuanced consideration to find the best balance between people and techniques.
If you would like to see ICRON in action, please contact us. If you have enjoyed this blog, you can find more content that you might enjoy here.